AI in Cancer Research - A Real Game Changer?
World Cancer Day: AI as a beacon of hope
Cancer is still the second most common cause of death in Germany. Despite research, there are still no effective therapies for some types of cancer. However, every four-week delay in treatment increases the risk of death by 6-13 percent. AI in cancer research can help.
Why Does the Use of AI in Cancer Research and Drug Development Make Sense?
Cancer remains one of the most significant challenges of modern medicine - at the latest, on World Cancer Day on February 4, this is brought home to us every year. For example, patients with particularly aggressive and difficult-to-treat cancers such as pancreatic cancer, liver cancer, and lung cancer often have low survival rates of well under five years in some cases (Santucci, C., et al., 2020). Rare cancers such as mesothelioma and certain central nervous system cancers are also challenging to treat (Jain, M., et al., 2024).
World Cancer Day was launched on February 4, 2000, during the World Summit Against Cancer for the New Millennium in Paris. On this day, the Paris Charter against Cancer was signed to promote research, prevention, and treatment of cancer worldwide. Since then, World Cancer Day has been celebrated annually on February 4 to raise cancer awareness and promote prevention measures. The International Union Against Cancer (UICC) takes the lead in organizing World Cancer Day.
However, the development of new drugs is expensive and time-consuming. Artificial Intelligence (AI) offers a promising opportunity to accelerate and optimize this process in cancer research (Wenz & Ebener, 2024). This brief selection of application examples alone clearly shows the potential behind this:
1. Revolutionizing Drug Development and Cancer Research Through AI and Other Advanced Computing Technology
Developing effective therapies is often challenging. One promising approach that can help researchers is using Artificial Intelligence (AI) in drug development. Here, advanced IT supports the research and development of new therapeutic strategies. The wide range of applications that AI offers in cancer research makes it a real game changer (Lotter, W., et al., 2024).
From research to preclinical and clinical studies, AI is opening up new opportunities for various application areas to make cancer research and drug development more efficient. For example, the use of AI in pharmaceutical and cancer research contributes significantly to optimizing therapies, detecting cancer earlier, and shortening development times for new drugs (Serrano, D. R.; et al., 2024).
2. Increasing Efficiency Through AI in Preclinical and Clinical Study Phases in Cancer Research and Drug Development
AI can also provide valuable services for preclinical and clinical cancer research and drug development studies. Both study phases are indispensable in developing new drugs but are time-consuming, cost-intensive, and often characterized by delays. According to an industry report of the vfa, the average time from clinical development to drug approval is 10.5 years - and only 8 percent of drugs make it there. Clinical development itself consumes as much as 50 percent of the total development costs of a drug. With AI, cancer research can tackle these challenges and thus significantly increase studies' efficiency and informative value (Viswa, C. A., et al., 2024). The following scenarios are conceivable, for example:
Support through computational drug discovery in the early phases of drug development
The classic drug development process begins with identifying a target molecule that plays a key role in a disease and ends with lead optimization, ensuring that the drug meets GLP regulations and FDA (Food and Drug Administration) requirements (Patheon, 2023). AI-supported technologies make it possible to predict the three-dimensional structure of proteins accurately. This opens up a deeper understanding of the molecular mechanisms of cancer cells and reveals potential drug targets (Jumper, J., et al., 2021). By rapidly analyzing vast data, AI supports a data-driven "fail-fast" approach that allows early screening of unpromising molecular candidates (Paul, D., et al., 2021). This type of AI in cancer research is also called "in silico", as the calculations and simulations are fully computer-aided (AI in Clinical Trials: Optimizing Study Design for Better Results.). Sovereign AI solutions ensure maximum data security and precise information processing.
Computational models and simulations can significantly accelerate the identification and optimization of potential active ingredients, reducing the time and resources required and the number of in vitro experiments needed. AI-supported molecular modeling benefits from high data quality and data standardization. This ensures more precise predictions and makes it possible to fully exploit the potential of technologies such as machine learning or customized workflows. Flexible AI services also enable research teams to react quickly to new requirements.
In the preclinical study phase of drug and cancer research, AI models can be used to predict the success of potential drugs at an early stage and avoid failures. At best, this would make it possible to reduce animal testing to a minimum. The analysis of toxicological profiles of known substances allows tests to be further optimized (Ghosh, Choudhary, & Medhi, 2024).
Literature research and large language models
A comprehensive analysis of scientific publications is essential in the search for suitable therapies. However, the sheer volume of such work makes manual research unmanageable and time-consuming. This is where Large Language Models (LLM) come into play, which can search through millions of scientific texts, automatically extract relevant information, and recognize correlations faster and more precisely than humans. For example, AI-supported bots sift through relevant studies on a particular type of cancer, provide a detailed overview of the latest research findings, and identify potential therapeutic targets.
Also, AI makes it possible to partially or even fully automate many steps in drug and cancer research. Certain technologies, such as Robotics Process Automation, facilitate the analysis of vast amounts of data, while Business Process Management allows existing to model processes and optimize them. As this eliminates time-consuming manual tasks, drug discovery and development becomes more efficient, significantly shortening the market time.
The clinical trial phases aim to validate new active substances' safety, efficacy, and dosage of new active substances. Here, too, AI can make a significant contribution to cancer research:
Patient recruitment and retention
AI in drug development and cancer research improves patient recruitment by analyzing large data sets and reducing recruitment time, which, according to the industry report, takes about one-third of the total study duration, by 20-40 percent (Hutson, 2024). Drop-outs in clinical trials, on the other hand, are problematic as they can affect the required sample size and thus the statistical power (Gyawali et al, Biases in study design, implementation, and data analysis that distort the appraisal of clinical benefit and ESMO-Magnitude of Clinical Benefit Scale ESMO-MCBS scoring). Optimized study designs and precise calculations are crucial to closing the gender data gap in medicine and achieving valid results (Lee & Wen, 2020). Generative AI optimizes study design, shortens the planning process by up to 50 percent, and increases precision through adaptive protocols. This reduces development times and improves patient care. Innovative methods such as collaborative drug delivery can also ensure patient safety when administering drugs (Gholap, A. D., et al., 2024).
Optimized study design
Artificial intelligence, particularly Generative AI (GenAI), is central to optimizing study design and planning. GenAI automatically analyzes large amounts of data, recognizes patterns, and provides data-based recommendations, shortening the planning process by up to 50 percent. Another advantage is precision: GenAI helps to focus studies on the right questions, clearly define endpoints, and efficiently calculate the sample size. Adaptive protocols based on real-time data also increase the flexibility and informative value of the studies. This reduces development times and ensures better patient care (AI in Clinical Trials: Optimizing Study Design for Better Results.).
Computational drug discovery refers to the use of computer-aided methods and technologies such as AI, machine learning and molecular modeling to accelerate the development of new active ingredients.
Collaborative Drug Delivery> focuses on the targeted administration of drugs using innovative technologies and personalized therapy systems. The aim is to maximize the effect and minimize side effects.
3. personalized medicine (precision medicine) through AI in cancer research
Artificial Intelligence plays a decisive role in precision medicine - i.e., personalized therapy - Artificial Intelligence plays a decisive role. The following scenarios are conceivable:
Early detection and diagnosis of cancer
Successful cancer treatment stands and falls with the early detection of the disease. AI algorithms can provide valuable services here by quickly and accurately evaluating medical images such as MRIs and CT scans. This allows tumors to be detected early, significantly increasing the chances of successful treatment (Bi, W. L., Hosny, A., Schabath, M. B., et al., 2019).
AI-supported antibody development in cancer research
AI makes a decisive contribution to developing antibodies, representing one of the most essential categories of biopharmaceutical therapeutics in cancer research. By analyzing the binding affinities and properties of the target proteins, antibodies can be developed faster and more precisely. AI thus reduces the time-consuming and cost-intensive in-vitro validation phase and increases the efficiency and precision of drug research, particularly in cancer and autoimmune therapy. Studies assume that AI can save 25-50 percent of costs when a drug reaches the clinical phase (Sobhani, D'Angelo, Pittacolo, Mondani, & Generali, 2024) (Nature, 2023).
Increasing the probability of success through AI in drug and cancer research
An additional advantage is that using AI in drug and cancer research significantly increases the probability of success in developing new therapies. Computational drug discovery enables precise prediction models based on a tumor's individual genetic and molecular characteristics. This makes it possible to identify the best molecule candidates more quickly and thus find the optimal active substance for treatment. Customized drugs can be used to develop targeted treatment strategies that promise a higher success rate in a shorter time (Jayatunga, Xie, Ruder, Schulze, & Meier, 2022).
Personalized medicine (precision medicine) through AI in cancer research
AI will also play a decisive role in precision medicine in the future by analyzing data from various sources. These include transcriptome data generated by next-generation sequencing (NGS), histological findings using imaging techniques, and patient histories based on traditional anamnesis. Based on this information, it is then possible to tailor treatment to specific patient groups or even individual patients (Dlamini, Z., et al., 2022).
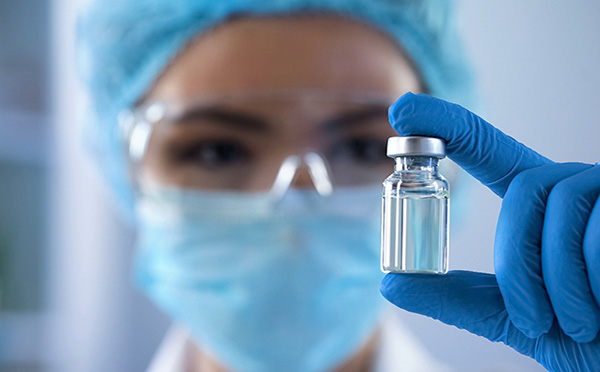
BioNTech, for example, is working on personalized mRNA vaccines to treat various cancers. The company uses AI to identify the best neoantigens for its mRNA vaccines. Neoantigens are specific proteins that occur on the surface of cancer cells and stimulate the immune system to fight cancer cells (BioNTech, 2024).
How Can AI Be Meaningfully Integrated Into Cancer Research and Drug Development?
Therefore, AI is likely to play an increasingly important role in drug and cancer research. Modern data management solutions enable the secure provision of data. The prerequisite for this is a well-thought-out Data strategy, which forms the basis for the efficient use of this information. Scalable AI solutions, in turn, make it possible to flexibly expand the models and adapt them to new challenges.
The following tools and strategies, in particular, offer valuable approaches and can significantly support the successful development of new therapies:
High-performance computing and cloud computing for drug research
Scalable high-performance computing solutions enable the pharmaceutical company to carry out complex simulations efficiently. Flexible cloud computing options allow them to add additional computing capacity as needed to efficiently handle peak loads, such as those that arise during virtual screening processes.
AI services for drug and cancer research
Using AI and machine learning in cancer research allows research-based pharmaceutical and biotech companies to gain precise insights for drug development. Integrating these technologies should be a central component of the entire development process—from data preparation to model training and practical implementation. There should also be a particular focus on compliance with industry-specific standards and individual requirements. It is helpful here to involve a partner with the relevant expertise who ensures compliance with all specifications through thorough code reviews.
Data management and integration for AI-supported drug and cancer research
Robust systems enable fast and secure consolidation of big data from omics studies and external data sources. AI for cancer research requires a high degree of flexibility in scaling and strict security requirements that ensure the best protection of research data at all times. It is essential that the data centers used not only meet high security and data protection standards, but can also prove this through comprehensive certifications. A Security Operation Center (SOC) also ensures that the confidentiality and integrity of the data are guaranteed at all times through continuous monitoring.
Cybersecurity and compliance
Customized cybersecurity solutions that are specifically tailored to the pharmaceutical industry and protect sensitive research data from cyber threats are constructive. Ideally, the service provider not only has the required IT expertise and knowledge of biological processes but also ensures compliance through access controls, data encryption, and regular security audits. This allows both industry-specific regulations and internal guidelines to be met while developing individual security strategies for biological data processing.
Trusted provider: Trusted Third Party
The data that pharmaceutical companies, biotech companies, and research institutions work with is particularly confidential and proprietary. Ensuring a high standard of protection here is, therefore, a must. Consequently, relying on a trustworthy partner (trusted third party) makes sense. This partner takes care of the secure integration of data and algorithms and provides a platform that enables the secure data exchange without compromising confidentiality. Encrypted data management, homomorphic encryption, and differential privacy guarantee data security. By integrating data from different sources on a secure platform, the trusted provider supports the development of innovative approaches in drug and cancer research (such as AI-powered bots), helping to accelerate the entire development process.
Challenges and Pitfalls of AI in Cancer Research and Drug Development
Despite all the benefits that AI brings to pharmaceutical and cancer research, it also poses certain challenges and risks that require special attention:
Privacy and security in AI-protected drug and cancer research
Healthcare data processing requires the highest standards of data protection and security. While anonymizing data is often considered the ideal solution, it also ensures that important details are lost, which affects the accuracy of AI analyses and leads to less accurate diagnoses. Especially when pseudonymization or anonymization is not an option, such as in epidemiological studies, it is important for researchers to store their data in-house wherever possible or to rely on a provider that offers certified and secure data environments.
Risk management and the "black box" problem
The new challenges of the AI era require a modernized approach to risk management in the pharmaceutical industry. Traditional control mechanisms are too slow to keep pace with the dynamics of modern technologies and flexible working methods. Instead, risks need to be managed proactively and flexibly. An expert partner provides the experience and AI-specific knowledge required to identify sources of error and thus minimize risks. Therefore, robust risk management is crucial for patient safety. By generating real-time data, AI-based systems used in drug and cancer research, for example, can recognize risk patterns at an early stage, leading to faster response times.
In addition, many AI systems act like a "black box" in which the decision-making processes are not transparent. This lack of transparency in AI could be problematic in drug and cancer research. This is because it is essential for researchers and regulatory authorities to be able to understand how decisions are made. This raises the key question: Is the use case subject to GxP requirements, and is it possible to validate it? This is where it is particularly valuable to have a trustworthy partner who can support both the latest technologies and the entire pharmaceutical industry value chain.
Another problem is the much-discussed phenomenon of hallucinations in machine-learning models. However, this can be reduced by careful selection and integration of high-quality data, a robust validation concept, and regular review and updating of the models. In addition, using explanatory models helps to better understand the decision-making processes and identify sources of error. A strong partner with experience in industry-specific best practice approaches can also provide support.
Pharmaceutical companies, biotech companies, and research institutions should rely on a solid digitization roadmap and a well-thought-out AI strategy. Adherence to strict data governance standards is just as important as building a robust AI framework that includes the planning, training, and hosting of ready-made solutions. This enables the company to ensure the traceability of AI decisions on the one hand and prevent potential risks on the other.
Conclusion: AI in Drug and Cancer Research Is Not a Dream of the Future
AI offers groundbreaking opportunities for drug and cancer research by taking the possibilities of diagnostics, therapy, and research to a whole new level. From molecule modeling to patient recruitment and approval, AI-powered solutions can speed up processes, reduce costs, and drive the development of personalized therapies. To exploit this potential, the high quality and quantity of data used to train the AI algorithms are just as important as a high-performance and scalable IT infrastructure and processes that include clear guidelines and new risk culture. It is therefore advisable to work with a partner who can manage the entire workflow, from developing an AI strategy about data Governance>> and robust AI frameworks through process optimization and modern data management to providing comprehensive solutions for innovative cancer research. If all these aspects come together, we can celebrate significant milestones in oncology on future World Cancer Days.
-
Bibliography
AI in Clinical Trials: Optimizing Study Design for Better Results. (no date). Retrieved from Maxis Clinical: https://www.maxisclinical.com/insights/blog/ai-in-clinical-trials-optimizing-study-design-for-better-results/
Bi, W. L., Hosny, A., Schabath, M. B., et al. (2019). Artificial intelligence in cancer imaging: Clinical challenges and applications. CA Cancer Journal for Clinicians, 69 (2), pp. 127-157. doi:10.3322/caac.21552.
BioNTech (ed.). (November 14, 2024). Innovation Series R&D Day 2024. Retrieved from https://investors.biontech.de/system/files-encrypted/nasdaq_kms/assets/2024/11/14/9-45-16/Innovation%2BSeries%2BR%26D%2BDay%2B2024.pdf
Dlamini, Z., et al. (2022). AI and precision oncology in clinical cancer genomics: From prevention to targeted cancer therapies-An outcomes-based patient care. Informatics in Medicine Unlocked, 31, p. 100965.
Gholap, A. D., et al. (2024). Advances in artificial intelligence for drug delivery and development: A comprehensive review. Computers in Biology and Medicine, 178, p. 108702 . doi:10.1016/j.compbiomed.2024.108702
Ghosh, A., Choudhary, G., & Medhi, B. (2024). The pivotal role of artificial intelligence in enhancing experimental animal model research: A machine learning perspective. Indian Journal of Pharmacology, 56(1), pp. 1-3. doi:DOI: 10.4103/ijp.ijp_81_24
Hanna, T. P., King, W., Thibodeau, S., Jalink, M., Paulin, G., Harvey-Jones, E., . . Aggarwal, A. (November 4, 2020). Mortality due to cancer treatment delay: Systematic review and meta-analysis. The BMJ, 371. doi:https://doi.org/10.1136/bmj.m4087.
https://www.worldcancerday.org/about/faqs. (no date).
Hutson, M. (2024). How AI is being used to accelerate clinical trials. Nature, 627(8003), pp. S2-S5. doi:10.1038/d41586-024-00753-x.
Jain, M., et al. (September 30, 2024). Malignant pleural mesothelioma: A comprehensive review. Journal of Clinical Medicine, 13(19), p. 5837. doi:10.3390/jcm13195837
Jayatunga, M. K., Xie, W., Ruder, L., Schulze, U., & Meier, C. (2022). AI in small-molecule drug discovery: A coming wave? Nature Reviews Drug Discovery, 21, pp. 175-176.
Jumper, J., et al. (2021). Highly accurate protein structure prediction with AlphaFold. Nature, 596(7873), pp. 583-589. doi:10.1038/s41586-021-03819-2
Lee, E., & Wen, P. (2020). Gender and sex disparity in cancer trials. ESMO Open, 5(Supplement 4), p. e000773 . doi:10.1136/esmoopen-2020-000773
Lotter, W., et al. (2024). Artificial intelligence in oncology: Current landscape, challenges, and future directions. Cancer Discovery, 14 (5), pp. 711-726. doi:DOI: 10.1158/2159-8290.CD-23-1199.
Nature. ( 2023). AI's potential to accelerate drug discovery needs a reality check. Nature, 622(7982), p. 217. doi:10.1038/d41586-023-03172-6
Patheon (ed.). (October 2023). Exploring the five phases of drug development.
Paul, D., et al. (2021). Artificial intelligence in drug discovery and development. Drug Discovery Today, 26(1), pp. 80-93. doi:DOI: 10.1016/j.drudis.2020.10.010
Santucci, C., et al. (2020). Progress in cancer mortality, incidence, and survival: A global overview. Eur J Cancer Prev, 29(5), pp. 367-381. doi:10.1097/CEJ.0000000000000594.
Serrano, D. R.; et al. (2024). Artificial Intelligence (AI) Applications in Drug Discovery and Drug Delivery: Revolutionizing Personalized Medicine. Pharmaceutics, 16(10), p. 1328. doi:10.3390/pharmaceutics16101328.
Shand, J., Stovold, E., Goulding, L. et al. (November 1, 2024). Cancer care treatment attrition in adults: Measurement approaches and inequities in patient dropout rates - a rapid review. BMC Cancer, 1345(24). doi:https://doi.org/10.1186.
Sobhani, N., D'Angelo, A., Pittacolo, M., Mondani, G., & Generali, D. (2024). Future AI will most likely predict antibody-drug conjugate response in oncology: A review and expert opinion. Cancers, 16, p. 3089. doi:DOI: 10.3390/cancers16013
Viswa, C. A., et al. (January 9, 2024). Generative AI in the pharmaceutical industry: Moving from hype to reality. (M. &. Company, ed.)
Wenz, F., & Ebener, S. (2024). Applications of artificial intelligence in oncology: opportunities, feasibility and regulatory challenges. - Journal. Oncology, 30, pp. 339-346. doi:10.1007/s00761-023-01428-4